Is most published research wrong?
Scientific research can be manipulated through p value and other hacks. Let's explore how.
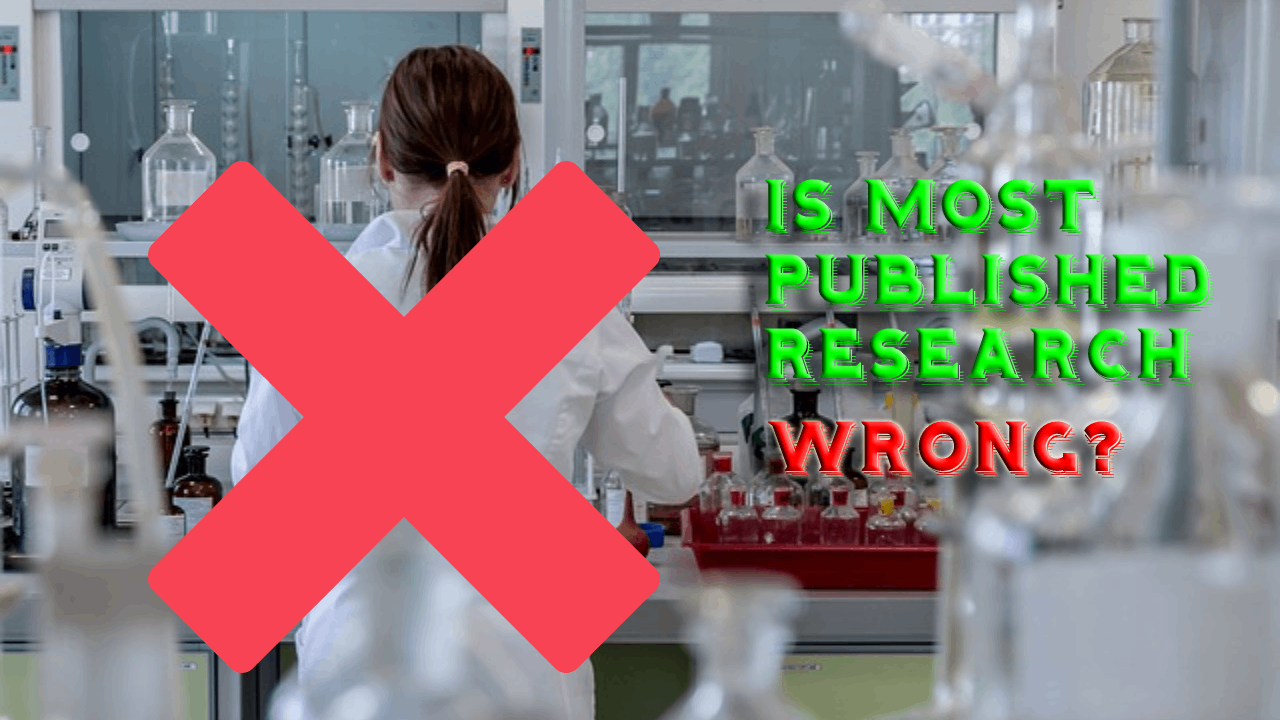
I recently watched a video from Veritasium. In the video, they explained why a large portion of the published scientific findings are wrong. This might get a bit technical, but I will try to use the simple language to explain it. So tighten up the seat belts and let’s see why it is the case.
What is p-value
Let’s say that we are trying to prove a hypothesis. For example, we suspect that an octopus can predict the soccer match results (sounds familiar?). We now want to prove it. We need to conduct experiments and show that the experiment results are confirming the hypothesis.
In our experiment, we show two boxes containing food to the octopus decorated with the flags of the teams playing. From whichever box, octopus eats from first, will be considered the octopus’ prediction.
The null hypothesis here is that the octopus can’t predict the future. That is, there is no relationship between the soccer match results and the box from with the octopus eats from first. So, about half of the predictions will be wrong. But what if more than half of the predictions (say 8 out of 14, i.e., 57%) are correct? Can we confidently say that the octopus can predict the future?
One way of justifying the statistical significance is to use the ‘P-value’. It is the probability of seeing the obtained results assuming that the null hypothesis is correct. An observed experiment result is considered ‘publishable’ if the p-value for that is less than 0.05. That is, assuming the null hypothesis is correct, the probability of seeing that kind of result is less than 5%. In the above experiment, the p-value is about 0.4 (assuming the binomial distribution). That is, there is a 40% chance that the octopus can get 8 predictions correct even if it can’t see the future. So, the result is not really statistically significant for a publication.
But, this means that about 5% of the published work can be wrong. They just obtained the false results by chance. If the experiments were repeated multiple times, we will not see those results. This is a problem. The bigger problem is that 5% is a huge underestimation of false results. Why?
Prior distribution
Let’s say that there are about 1000 hypotheses being tested by scientists. Let’s assume that about 10% of them (that is, 100) are actually correct. But no one knows which 100 are correct. Out of the actual 100 true hypotheses, let’s say that scientists verified 80 of them correctly (True positives) and couldn’t prove the other 20 because of some errors in the experiment (False negatives).
Now considered the remaining 900 false hypotheses. The 5% of those (that is, 45) will be incorrectly proved (because of the p-value). These are the false positives. The rest of them will be correctly disproved (True negatives). But most journals rarely publish null results. Let’s say about 20 of those true negatives are published.
So, overall, we have published all the 80 true positives, 45 false positives and 20 true negatives. So, about one third of the published results are wrong (False positives). The actual numbers can be worse if the prior distribution of correct and wrong hypothesis is more skewed.
P-hacking
By the way, Paul, the octopus, predicted 12 out of 14 results correctly. This corresponds to the p-value about 0.006. This is statistically significant as per the p-value. But is it? The number of trials is way too small to justify the statistical significance.
There are many ways in which p-value can be manipulated. Scientists can design experiments in such a way that the likelihood of seeing the false proofs can be increased. Or present the data in such a way that it shows statistical significance even when there is none. This is called p-hacking. Even if the scientists are not trying to hack the p values, they might be biased towards a particular types of results and that can sometimes affect the way in which the experiment results are published.
Reproducibility
So, if the problem is just about p-value, can’t we fix it by attempting to reproduce the results? If the hypothesis was proved by a fluke, it will most likely not be reproduced when we repeat the experiment. But, reproducing the results is not as easy as it sounds. The paper authors often fail to provide sufficient details for reproducing their experiment results. So, if other scientists fail to reproduce the results, the journal will reject their paper by saying that they didn’t do the experiment correctly.
On top of that, journals are more interested in publishing counterintuitive hypotheses. The reproducibility studies are not that interesting. So, not many scientists are motivated to reproduce the already published experiment results. They would rather prefer to test a new hypothesis.
What to trust now
Well, however flawed the scientific research publications are, the other ways of verifying the truth are worse. So, we should still be using the scientific research for verifying the truths. But at the same time, we must acknowledge that the published research is not always right. We should try to address the underlying issues mentioned above. Fortunately, scientific community is moving towards solving them. The procedures are analyzed more carefully. The reproducibility studies and publication of null results are being incentivized. Etc. We will get there some day!
Interesting read: “Why Most Published Research Findings Are False” by John P. A. Ioannidis
My favorites
Video: What special relativity feels like (action lab)
Quote: “The only thing that interferes with my learning is my education.” — ALBERT EINSTEIN, retrieved from The millionaire fastlane by M. J. DeMarco